Data science is evolving rapidly, driven by advancements in technology and the increasing availability of data. As we look ahead to the next decade, several trends and topics are expected to shape the field. In this article, we will explore the key data science topics in the next 10 years, covering advancements in machine learning, big data, ethical considerations, and more.
Data Science Topics in the Next 10 years
1. Machine Learning and Artificial Intelligence
Advanced Machine Learning Models
One of the most significant data science topics in the next 10 years is the development of advanced machine learning models. These models will become more sophisticated, capable of understanding complex patterns and making accurate predictions. Techniques such as deep learning, reinforcement learning, and generative adversarial networks (GANs) will continue to evolve, enabling applications in areas like natural language processing, image recognition, and autonomous systems.
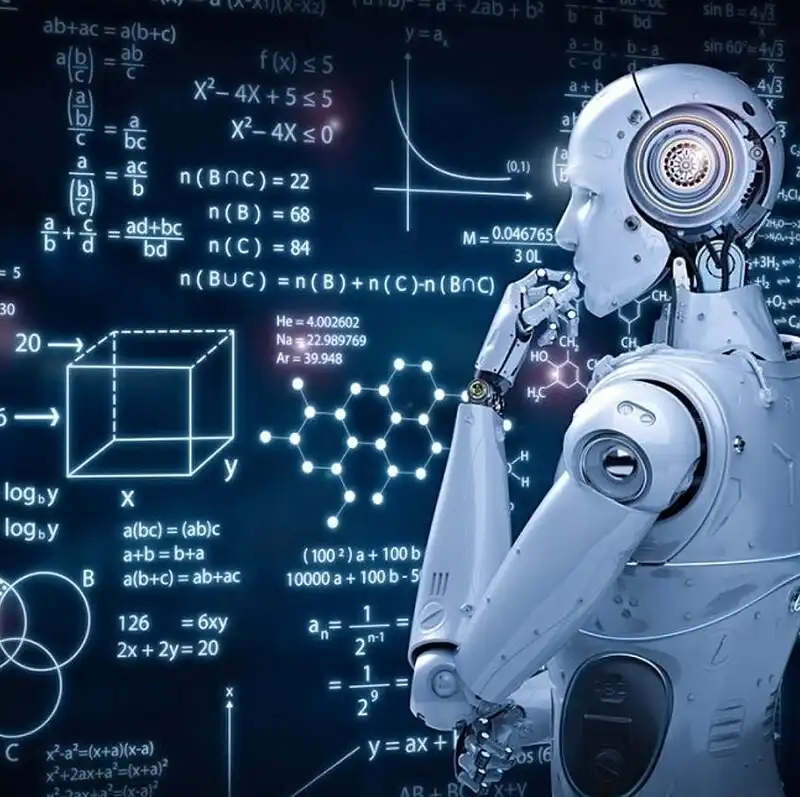
Explainable AI
As AI systems become more integrated into our daily lives, the need for explainable AI (XAI) will grow. Explainable AI aims to make machine learning models more transparent and understandable to humans. This will be crucial for building trust in AI systems, especially in critical applications like healthcare, finance, and autonomous driving.
2. Big Data and Data Management
Data Integration and Interoperability
With the explosion of data from various sources, integrating and managing this data efficiently will be a major focus. Data integration and interoperability will be key data science topics in the next 10 years. Researchers and practitioners will develop new methods and tools to seamlessly combine data from different sources, formats, and systems, ensuring that organizations can leverage their data assets effectively.
Real-Time Data Processing
The demand for real-time data processing is expected to rise significantly. Businesses will require systems that can analyze data as it is generated, enabling instant decision-making. This will lead to advancements in streaming data technologies, edge computing, and in-memory databases, allowing for faster and more efficient data processing.
3. Ethical and Social Implications
Data Privacy and Security
As data becomes more pervasive, ensuring data privacy and security will be paramount. Data science topics in the next 10 years will include developing robust techniques for protecting sensitive information and preventing data breaches. This will involve advancements in encryption, anonymization, and secure data sharing protocols.
Bias and Fairness in AI
Addressing bias and ensuring fairness in AI systems will be crucial. Data scientists will need to develop methods to detect and mitigate biases in datasets and algorithms. This will involve creating diverse and representative datasets, as well as implementing fairness-aware machine learning techniques.
4. Industry-Specific Applications
Healthcare
In the healthcare sector, data science will play a transformative role. Predictive analytics, personalized medicine, and genomics are expected to be major data science topics in the next 10 years. Data-driven approaches will enable early disease detection, optimized treatment plans, and improved patient outcomes. That’s why Biological Data Scientist’s or Bioinformatician’s salary keep on increasing worldwide.
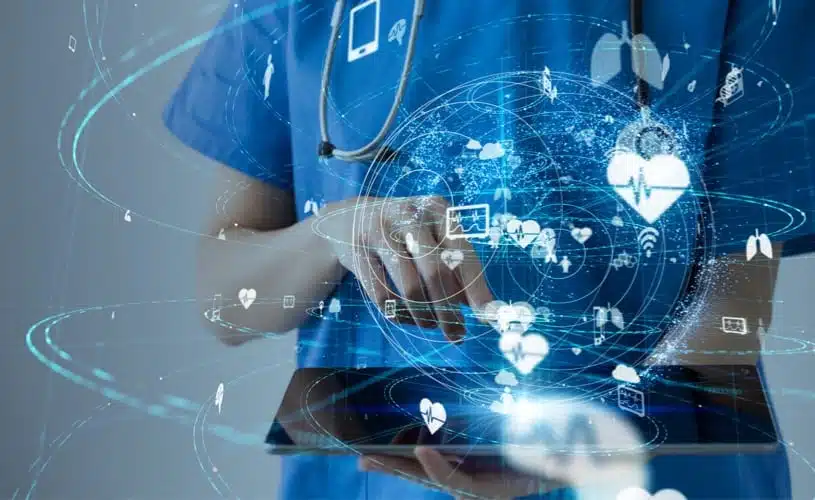
Finance
Data science will continue to revolutionize the finance industry. Fraud detection, algorithmic trading, and risk management will be key areas of focus. Advanced analytics and machine learning models will help financial institutions make better decisions, detect anomalies, and enhance customer experiences.
5. Emerging Technologies
Quantum Computing
Quantum computing has the potential to revolutionize data science by solving complex problems that are currently infeasible for classical computers. Over the next decade, we will see significant advancements in quantum algorithms and their applications in data science. Quantum computing will enable breakthroughs in optimization, cryptography, and machine learning.
Internet of Things (IoT)
The proliferation of IoT devices will generate massive amounts of data. Managing and analyzing this data will be a major data science topic in the next 10 years. Data scientists will develop new methods to handle the scale, velocity, and variety of IoT data, enabling applications in smart cities, industrial automation, and healthcare.
6. Educational and Workforce Implications
Data Science Education
As the demand for data scientists grows, so will the need for effective education and training programs. Universities and institutions will need to update their curricula to cover emerging data science topics in the next 10 years. This will include hands-on training with the latest tools and technologies, as well as interdisciplinary approaches that combine data science with domain-specific knowledge.
Continuous Learning and Upskilling
Given the fast-paced nature of the field, continuous learning and upskilling will be essential for data science professionals. Online courses, bootcamps, and certifications will play a crucial role in helping individuals stay updated with the latest advancements. Employers will also need to invest in training programs to ensure their workforce remains competitive.
7. Collaboration and Open Science
Collaborative Research
Collaboration between academia, industry, and government will be vital for advancing data science topics in the next 10 years. Joint research initiatives and public-private partnerships will drive innovation and ensure that data science solutions address real-world challenges.
Open Data and Open Source
The open science movement will continue to grow, promoting the sharing of data, models, and tools. Open data initiatives will provide researchers with access to valuable datasets, while open-source software will facilitate the development and dissemination of cutting-edge techniques. This collaborative approach will accelerate progress and democratize access to data science resources.
8. Environmental and Sustainability Applications
Climate Change Analysis
Data science will play a crucial role in addressing environmental challenges. Climate change analysis, for instance, will be a significant focus. By analyzing vast amounts of climate data, scientists can better understand patterns, predict future changes, and develop strategies to mitigate the impact of climate change.
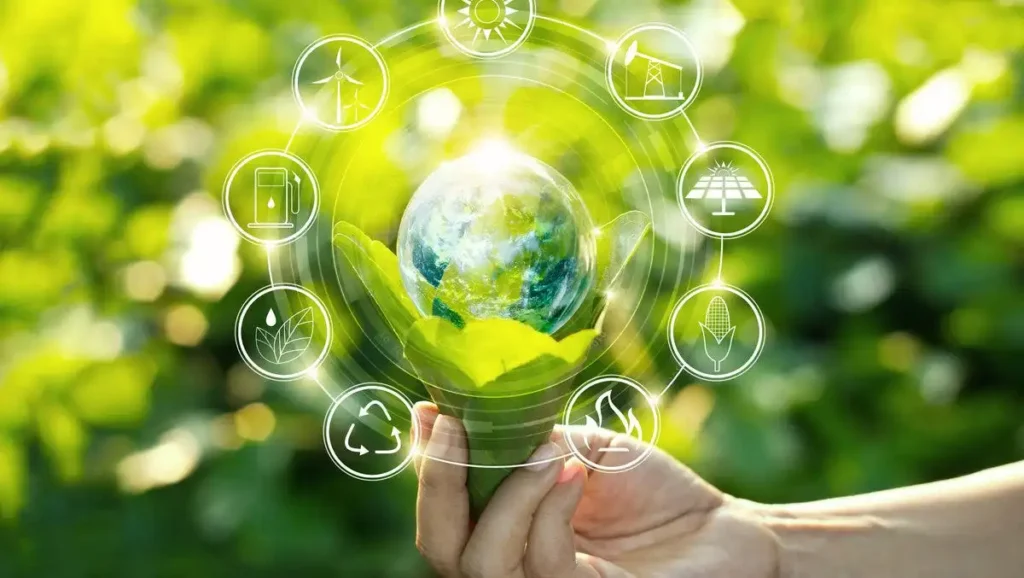
Sustainable Resource Management
Efficient management of natural resources will be another key data science topic in the next 10 years. Data-driven approaches will optimize the use of resources such as water, energy, and minerals. This will involve developing predictive models for resource consumption, monitoring environmental impacts, and implementing sustainable practices.
9. Human-Centric AI and User Experience
Human-AI Interaction
Improving the interaction between humans and AI systems will be a major focus. Human-centric AI aims to create systems that are intuitive, easy to use, and enhance human capabilities. This will involve advancements in natural language processing, computer vision, and user interface design to create seamless and effective interactions.
Personalization and User Experience
Data science will enable highly personalized experiences across various domains. In e-commerce, for example, data-driven personalization will enhance customer recommendations and improve satisfaction. Similarly, in education, personalized learning paths will cater to individual needs, improving learning outcomes.
10. Ethical AI Governance
Ethical Frameworks and Policies
As AI and data science become more integrated into society, the development of ethical frameworks and policies will be crucial. These frameworks will guide the responsible use of AI, ensuring that it aligns with societal values and norms. Policymakers, researchers, and industry leaders will need to collaborate to establish standards and regulations.
Transparency and Accountability
Ensuring transparency and accountability in AI systems will be essential for building trust. Data science topics in the next 10 years will include developing methods to audit and explain AI decisions, as well as mechanisms to hold organizations accountable for their AI practices. This will involve creating robust documentation, conducting regular audits, and establishing clear lines of responsibility.
Conclusion
The field of data science is poised for significant advancements in the next decade. From advanced machine learning models to ethical considerations, the data science topics in the next 10 years will shape our world in profound ways. By staying informed and adapting to these trends, data scientists can continue to drive innovation and make meaningful contributions to society.