The rapid development of artificial intelligence (AI) has significantly impacted various fields, including biotechnology and bioinformatics. AI tools are transforming how scientists conduct research, analyze data, and make discoveries. In this article, we will explore some of the latest AI tools for biotech and bioinformatics, their applications, pros and cons, availability showcasing their impact.
What are AI Tools for Biotech and Bioinformatics?
AI tools for biotech and bioinformatics are software and algorithms designed to perform tasks that traditionally required human intelligence. These tasks include pattern recognition, data analysis, prediction modeling, and decision-making. By automating these processes, AI tools enable researchers to analyze large datasets more efficiently, uncover hidden patterns, and make predictions that were previously impossible.
Latest AI Tools for Biotech and Bioinformatics
The field of AI in biotech and bioinformatics is constantly evolving, with new tools being developed to tackle emerging challenges. Here are some of the latest AI tools that are making waves in the industry:
1. OpenCRISPR-1
What It Does:
OpenCRISPR-1, developed by Profluent AI, is a groundbreaking, AI-designed CRISPR-Cas system. This open-source gene editor has been engineered to offer unparalleled precision and customizability in gene editing. Unlike traditional CRISPR systems that are naturally occurring, OpenCRISPR-1 is fully designed by artificial intelligence, allowing for optimized performance across various biological systems, including human, animal, and plant cells.
Pros:
- Enhanced Precision: Thanks to AI optimization, OpenCRISPR-1 significantly reduces off-target effects, making it one of the most accurate gene-editing tools available.
- Customizability: The tool features 400 sequence variations, giving researchers the flexibility to select the most effective option for their specific gene-editing needs.
- Open Source: OpenCRISPR-1 is freely available to the global scientific community, supporting widespread innovation and application in genetic research.
Cons:
- Ethical Concerns: The powerful capabilities and broad accessibility of OpenCRISPR-1 raise ethical questions, particularly regarding its potential use in human genetic modifications.
- Resource Intensive: Developing and using AI-generated tools like OpenCRISPR-1 require significant computational resources, which may limit access for smaller research facilities.
Availability:
OpenCRISPR-1 is available under an open-source license, allowing researchers and developers worldwide to utilize this advanced tool for a variety of gene-editing applications. Profluent AI’s decision to make this technology open-source aims to democratize access to cutting-edge gene-editing capabilities, thereby accelerating progress in the field of genetic research.
Learn More: OpenCRISPR-1
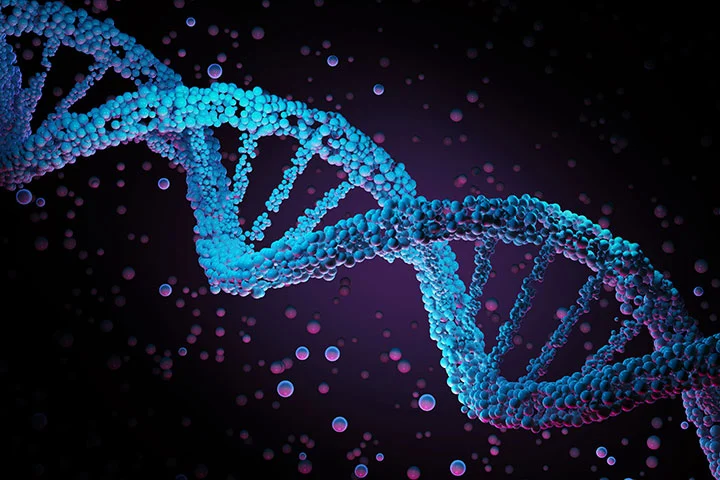
2. CRISPRoff
What It Does:
CRISPRoff is a gene-silencing technology that enables precise and reversible suppression of gene expression without altering the DNA sequence. Utilizing epigenetic modifications, it adds methyl groups to specific DNA regions to turn genes off effectively, allowing for controlled and heritable changes in gene activity that can be reversed as needed.
Pros:
- Reversible Gene Silencing: Allows genes to be turned off and subsequently reactivated, providing flexibility in studying gene functions.
- No DNA Cutting: Avoids double-strand breaks in DNA, reducing the risk of unintended mutations and genomic instability.
- Heritable Effects: Gene-silencing modifications can be passed down through cell divisions, making it useful for long-term studies.
Cons:
- Incomplete Silencing: May not achieve 100% suppression of target genes in all cases, potentially affecting experimental outcomes.
- Limited Understanding: The long-term effects and stability of epigenetic modifications induced by CRISPRoff are still being researched.
Availability:
CRISPRoff technology is available through collaborations with research institutions and biotech companies involved in gene-editing research. Some components and protocols may be accessible through scientific publications and may require licensing for commercial use.
Learn More: CRISPRoff
3. AlphaFold
What It Does:
AlphaFold, developed by DeepMind, predicts the 3D structures of proteins based on their amino acid sequences. It has made significant advances in structural biology by providing accurate predictions that were previously unattainable.
Pros:
- Breakthrough Accuracy: Provides near-experimental accuracy in protein structure prediction.
- Open Source: AlphaFold’s code and models are freely available, making it accessible to the global research community.
- Impactful: Has the potential to accelerate drug discovery and our understanding of biological processes.
Cons:
- High Demand: Computationally intensive, requiring significant resources to run at scale.
- Complex Interpretation: While AlphaFold predicts structures, interpreting these results for functional insights still requires expertise.
Availability:
AlphaFold is available as open-source software, with code and models accessible on GitHub.
Learn More: AlphaFold
4. DeepVariant
What It Does:
DeepVariant, developed by Google Health, uses deep learning to analyze genomic data and identify genetic variants with high accuracy. It is particularly effective in regions of the genome that are challenging to sequence.
Pros:
- Accuracy: Delivers highly accurate variant calls, even in difficult-to-sequence regions.
- Open Source: Available as open-source software, making it accessible to researchers worldwide.
- Continuous Updates: Regularly updated with improvements from the research community.
Cons:
- Computational Resources: Requires significant computational power, which may be a barrier for smaller labs.
- Complexity: Using DeepVariant requires expertise in bioinformatics and deep learning.
Availability:
DeepVariant is open-source and free to use, available on GitHub.
Learn More: DeepVariant
5. DeepCRISPR
What It Does:
DeepCRISPR is an AI-driven tool designed to predict the efficiency and specificity of guide RNA (gRNA) sequences used in CRISPR-Cas9 gene editing. Developed using deep learning algorithms, it analyzes vast genomic data to help researchers select the most effective and least off-target prone gRNA sequences for precise gene modifications.
Pros:
- Enhanced Accuracy: Provides highly accurate predictions for gRNA efficacy, reducing the likelihood of off-target effects.
- Time-Efficient: Streamlines the gRNA selection process by quickly analyzing and scoring numerous potential sequences.
- User-Friendly Interface: Offers an accessible platform that researchers can easily navigate without extensive computational expertise.
Cons:
- Data Dependency: Accuracy is influenced by the quality and diversity of input data, which may limit performance in less-studied genomes.
- Computational Resources: Running deep learning models can be resource-intensive, requiring powerful hardware for optimal performance.
Availability:
DeepCRISPR is available as an open-source tool, with code and documentation accessible on platforms like GitHub. Researchers can integrate it into their workflows and customize it according to specific project needs.
Learn More: DeepCRISPR
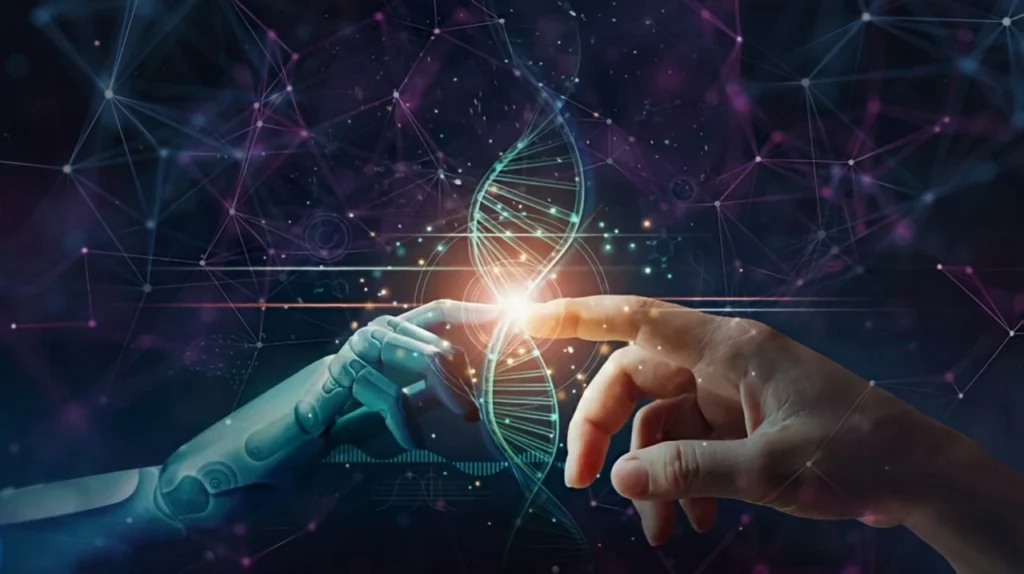
6. CellDIVE
What It Does:
CellDIVE is an AI-driven tool for single-cell RNA sequencing (scRNA-seq) analysis. It automates the identification of cell types and states, making it easier to study cellular diversity and function.
CellDIVE Applications:
- Cancer Progression Studies: CellDIVE is used to analyze the cellular composition of tumors, providing insights into how cancer progresses and develops resistance to treatment.
- Stem Cell Research: Researchers use CellDIVE to study stem cell differentiation, helping them understand how different cell types develop and function.
Pros:
- Automation: Reduces the time and effort required for scRNA-seq data analysis.
- High Accuracy: Accurately identifies and classifies different cell types.
- Scalability: Suitable for large datasets, making it ideal for studies involving complex tissues.
Cons:
- Computational Resources: Requires significant computational power, which may be a barrier for smaller labs.
- Cost: As a commercial tool, access to CellDIVE may be costly.
Availability:
CellDIVE is a commercial tool, available through partnerships and subscription models.
7. DeepChem
What It Does:
DeepChem is an open-source AI tool designed for drug discovery, materials science, and quantum chemistry. It provides machine learning models and tools for analyzing molecular data, making it a valuable resource for researchers in biotech and bioinformatics.
Pros:
- Open Source: Freely available, making it accessible to researchers worldwide.
- Versatile: Supports various applications, including drug discovery and materials science.
- Community Support: Backed by an active community of developers and researchers.
Cons:
- Complexity: Requires a good understanding of machine learning and chemistry to use effectively.
- Computational Resources: High computational requirements may be a barrier for some users.
Availability:
DeepChem is open-source and free to use.
Learn More: DeepChem
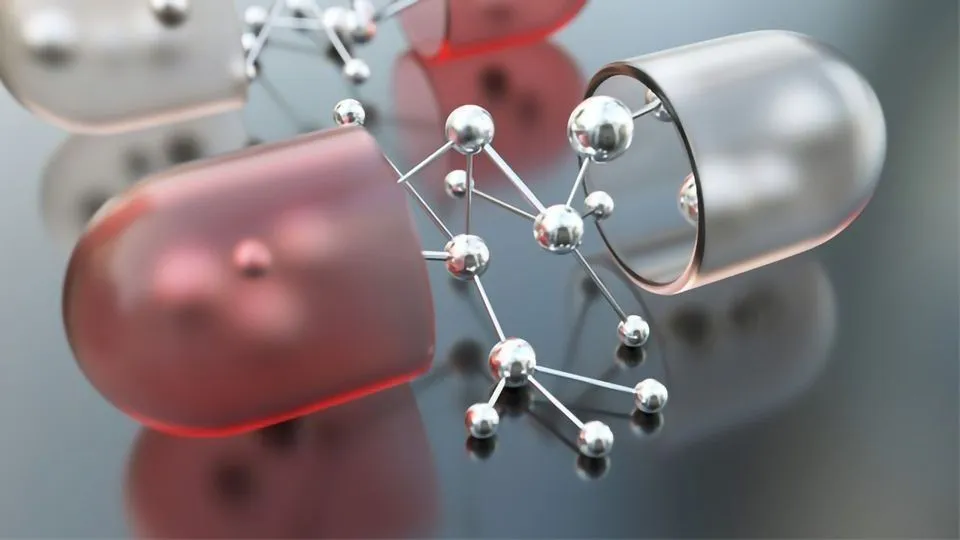
8. Tempus
What It Does:
Tempus is an AI-driven platform that analyzes clinical and molecular data to support personalized medicine. It leverages machine learning to predict how patients will respond to various cancer treatments, enabling personalized and more effective therapies.
Pros:
- Personalization: Helps tailor treatments to individual patients, improving outcomes.
- Comprehensive Data Integration: Analyzes a wide range of data, including genomic, clinical, and imaging data.
- Continuous Learning: The platform improves over time as it processes more data.
Cons:
- Data Privacy: Handling sensitive patient data raises privacy concerns.
- Cost: Tempus services are commercial and can be expensive for individual users or smaller clinics.
Availability:
Tempus is a commercial platform, and services are available through partnerships and subscription models.
Learn More: Tempus
9. Atomwise
What It Does:
Atomwise uses deep learning to predict how small molecules, such as potential drugs, will interact with proteins. This AI tool is widely used in drug discovery, enabling researchers to screen millions of compounds quickly and identify those most likely to be effective.
Pros:
- Speed: Accelerates the drug discovery process by quickly identifying potential drug candidates.
- Accuracy: Provides precise predictions of drug-target interactions.
- Scalability: Can screen vast libraries of compounds.
Cons:
- Cost: Atomwise is a commercial tool, so it may require significant investment, particularly for smaller research institutions.
- Data Dependency: The accuracy of predictions depends heavily on the quality of the input data.
Availability:
Atomwise is a commercial tool, and access requires payment. The company offers custom partnerships with pharmaceutical companies and research institutions.
Learn More: Atomwise
10. MetaPhlAn
What It Does:
MetaPhlAn (Metagenomic Phylogenetic Analysis) is an AI tool used for profiling microbial communities from metagenomic data. It identifies the composition of microbial populations in a sample, making it useful for studying the microbiome.
Pros:
- High Precision: Provides accurate taxonomic profiles of microbial communities.
- Open Source: Freely available, allowing wide access to researchers.
- Wide Applications: Used in various fields, including human microbiome studies, environmental research, and infectious disease research.
Cons:
- Complexity: Requires expertise in bioinformatics and metagenomics to use effectively.
- Data Dependency: The accuracy of results depends on the quality of the input data.
Availability:
MetaPhlAn is open-source and free to use.
Learn More: MetaPhlAn
Conclusion
AI tools for biotech and bioinformatics are driving innovation across multiple domains, from drug discovery to personalized medicine and evolutionary biology. These tools offer significant advantages, including speed, accuracy, and the ability to handle vast datasets. While some tools are open-source and freely available, others are commercial and require significant investment. Institutions and companies worldwide are already leveraging these AI tools to accelerate research and improve healthcare outcomes. As AI technology continues to advance, its role in biotech and bioinformatics will undoubtedly expand, offering new possibilities and solutions to some of the most pressing challenges in science and medicine.