Bivariate data is like a duo—a pair of pieces of information that go hand in hand. It’s like looking at two variables together to see if there’s a connection between them. Let’s delve into what bivariate data is all about, its types, characteristics, measures, and formulas. We’ll explore some fascinating examples from the biosciences realm, including healthcare, genomics, environmental science, clinical research, and pharmaceuticals.
What is Bivariate Data?
Bivariate data is a fancy term for saying we’re looking at two things at once. It’s like comparing apples and oranges—you’re interested in how they relate to each other. In statistics, we use bivariate data to see if there’s a connection or relationship between two variables.
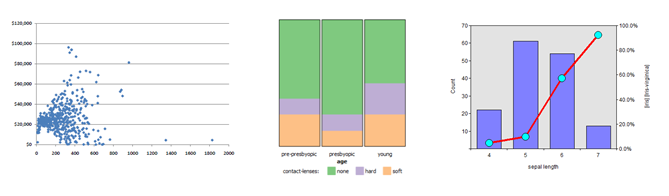
Types of Bivariate Data
- Positive Bivariate Data: When one variable increases, the other tends to increase as well. It’s like saying more sunshine leads to more happiness.
- Negative Bivariate Data: Here, when one variable increases, the other decreases. Think of it as saying more pollution leads to fewer trees.
Also learn about Bioinformatics vs Biostatistics; A 2024 Analysis of Biological Data Trends.
Characteristics of Bivariate Data
- Interdependence: The variables depend on each other in some way. For example, in healthcare, a patient’s weight might depend on their height.
- Scatterplot Representation: Bivariate data is often represented using scatterplots, where each point represents a pair of values for the two variables.
- Correlation: This tells us how closely the two variables are related. It ranges from -1 to 1. A correlation of 1 means a perfect positive relationship, -1 means a perfect negative relationship, and 0 means no relationship at all.
Bivariate Measure
The measure used to assess the relationship between two variables is called correlation. It helps us understand how changes in one variable are associated with changes in another.
Bivariate Data Formula
Correlation is usually calculated using a formula known as Pearson’s correlation coefficient. It looks complicated, but it’s not so bad once you get the hang of it:
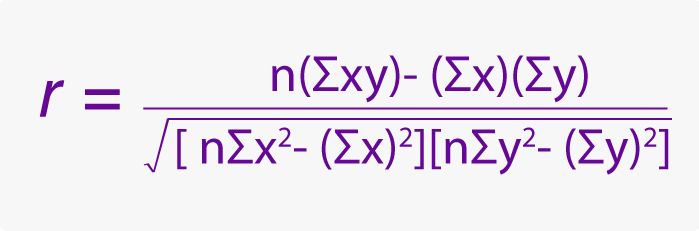
Where:
- n = number of data points
- x = values of the first variable
- y = values of the second variable
- ∑= summation (add up all the values)
- ∑xy = sum of the products of the paired values
- ∑x = sum of the first variable values
- ∑y = sum of the second variable values
- ∑x2 = sum of the squares of the first variable values
- ∑y2 = sum of the squares of the second variable values
The Concept Behind the Bivariate Data Formula:
Now, let’s delve into why this formula works. Pearson’s correlation coefficient measures the strength and direction of the linear relationship between two variables. Here’s how it does that:
Covariance:
The numerator of the formula, n(∑xy)−(∑x)(∑y), represents the covariance between the two variables. Covariance tells us how much two variables vary together. If the variables tend to increase or decrease together, the covariance will be positive; if one variable tends to increase as the other decreases, the covariance will be negative.
Standard Deviations:
The denominator of the formula involves the standard deviations of each variable. Standard deviation is a measure of how spread out the values of a variable are. Dividing the covariance by the product of the standard deviations standardizes the measure, giving us the correlation coefficient.
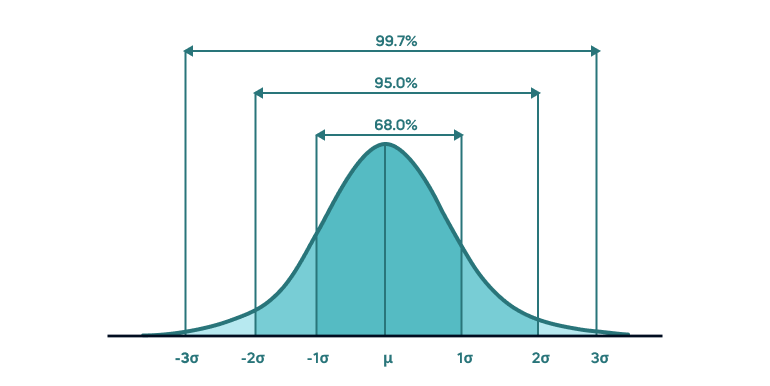
Normalization:
Dividing the covariance by the product of the standard deviations normalizes the measure, ensuring that the correlation coefficient r falls within the range of -1 to 1. This allows us to compare the strength and direction of the relationship between different pairs of variables, regardless of their units or scales.
Also explore Bivariate Analysis in Biological Data Science: Theory, Tools, and Practical Use Cases.
Examples of Bivariate Data in Biosciences
1. Healthcare: Body Mass Index (BMI) vs. Blood Pressure
Researchers study how BMI (body mass index) relates to blood pressure. They collect data on both BMI and blood pressure from a group of patients. By analyzing this bivariate data, they can see if there’s a correlation between high BMI and high blood pressure. Understanding this relationship helps in managing cardiovascular health.
2. Genomics: Gene Expression vs. Disease Susceptibility
In genomics, scientists analyze how gene expression levels relate to the susceptibility to certain diseases. By collecting bivariate data on gene expression and disease status across different individuals, they can identify genes that might play a role in disease development. This understanding contributes to personalized medicine and targeted therapies.
3. Environmental Science: Pollution Levels vs. Respiratory Illness
Environmental scientists examine how pollution levels affect respiratory illness rates in a community. By gathering bivariate data on pollution levels (such as air quality measurements) and the incidence of respiratory illnesses (such as asthma or bronchitis cases), they can assess the impact of environmental factors on public health. This information guides policies aimed at reducing pollution and protecting public health.
4. Clinical Research: Drug Dosage vs. Treatment Efficacy
Clinical researchers investigate the relationship between drug dosage and treatment efficacy for various medical conditions. By collecting bivariate data on the dosage of a medication administered to patients and their corresponding treatment outcomes, they can determine the optimal dosage that maximizes effectiveness while minimizing side effects. This research helps improve patient care and drug development processes.
5. Pharmaceuticals: Drug Concentration vs. Toxicity
Pharmaceutical companies conduct studies to assess the relationship between drug concentration levels in the body and the occurrence of adverse effects or toxicity. By analyzing bivariate data on drug concentrations measured in patients’ blood or tissues and any observed toxicity symptoms, they can establish safe dosage ranges for medications and identify potential safety concerns. This knowledge informs drug labeling, prescribing guidelines, and regulatory decisions to ensure patient safety.
Learn about 8 Data Science Portfolio Projects in Healthcare and Genomics; Step by Step Guidance and Resources
Conclusion
Bivariate data analysis is a powerful tool in various biosciences fields, allowing researchers to explore relationships between two variables and draw meaningful insights. Whether it’s understanding disease mechanisms, optimizing treatments, or safeguarding public health and the environment, bivariate data analysis plays a crucial role in advancing scientific knowledge and improving outcomes in the biosciences domain. So next time you’re faced with a bunch of data, don’t forget to look at it bivariately—you might uncover some fascinating connections!
Know more about 5 Top Statistical Programming Languages and Software for Biological Data Science.