The intersection of neuroscience and data science represents an intriguing frontier where the study of the brain meets the power of data analytics. By integrating principles from both fields, this interdisciplinary approach offers a unique opportunity to understand brain and revolutionize our understanding of neurological and psychiatric disorders.
In this article, let’s explore in detail how these seemingly disparate fields interact and complement each other in surprising ways.
What is Neuroscience?
Neuroscience is all about exploring everything about brain – how it works, how it’s structured, and how it influences our thoughts, feelings, and behaviors. Researchers in this field use a variety of tools and techniques to study the brain, from brain imaging scans to experiments with lab animals.
What is Data Science?
Data science, on the other hand, is all about making sense of data. It involves collecting, organizing, and analyzing large sets of information to uncover patterns, trends, and insights. Data scientists use a range of techniques, including statistics, machine learning, and artificial intelligence, to extract valuable knowledge from data.
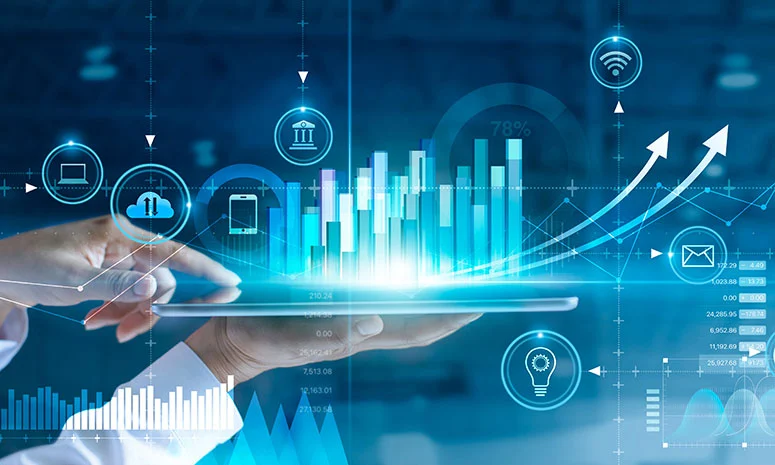
Where do Neuroscience and Data Science Correlate?
1. Brain Imaging
One area where neuroscience and data science intersect is brain imaging. Techniques like fMRI (functional Magnetic Resonance Imaging) allow researchers to capture detailed images of the brain in action. These images produce vast amounts of data, which data scientists can then analyze to understand patterns of brain activity associated with specific tasks or conditions.
2. Pattern Recognition
Data science excels at pattern recognition – identifying similarities and differences in data that might not be apparent to the naked eye. In neuroscience, this means using algorithms to analyze brain imaging data and identify patterns of activity associated with different thoughts, emotions, or behaviors. This can lead to new insights into how the brain works and how it can be affected by various factors.
3. Predictive Modeling
Another way neuroscience and data science interact is through predictive modeling. By analyzing large datasets of brain imaging data, researchers can develop models that predict how the brain will respond in different situations. For example, they might predict how a particular individual will respond to a certain stimulus or how a certain brain region will behave under specific conditions.
4. Clinical Applications
The interaction between neuroscience and data science has profound implications for clinical practice. By combining data from brain imaging scans with other types of clinical data, researchers can develop more accurate diagnostic tools for neurological and psychiatric disorders. They can also use predictive modeling to identify individuals at risk of developing these disorders and intervene early to prevent or mitigate their effects.
Neuroscience and Data Science Interaction Real World Examples
1. Brain-Computer Interfaces (BCIs)
BCIs are devices that translate brain activity into commands that control external devices, such as computers or prosthetic limbs. Data science plays a crucial role in developing algorithms that interpret neural signals recorded by BCIs, allowing users to interact with technology using their thoughts. These interfaces have applications in assistive technology for individuals with disabilities and in neurorehabilitation.
2. Neuroimaging Data Analysis
Neuroimaging techniques like fMRI and EEG generate large amounts of data that require sophisticated analysis. Data science methods, such as machine learning algorithms, are used to analyze neuroimaging data and identify patterns associated with different brain states, cognitive functions, or neurological disorders. This helps researchers gain insights into brain function and dysfunction.
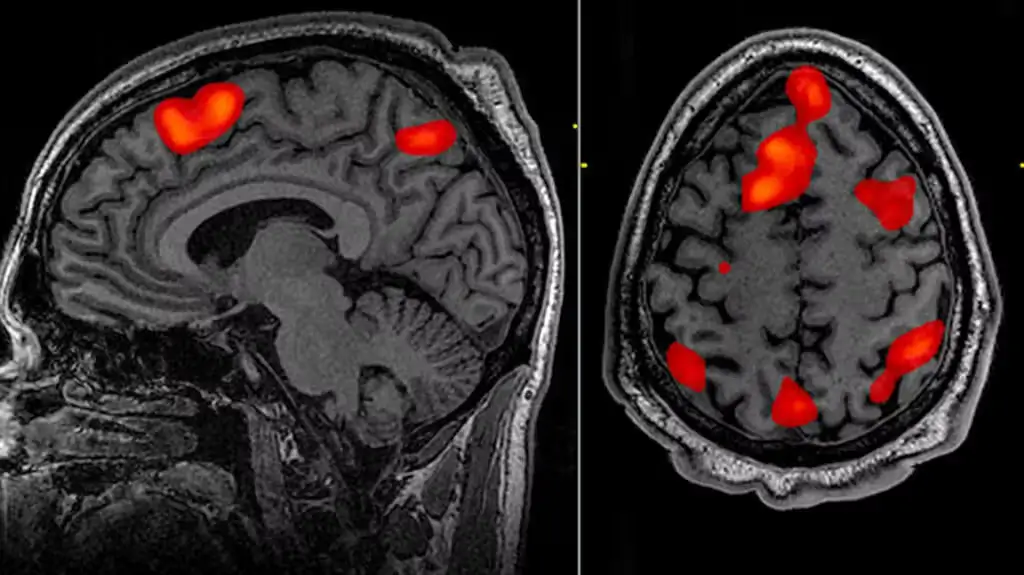
3. Predictive Modeling for Mental Health
Data science techniques are applied to large datasets of clinical and neuroimaging data to develop predictive models for mental health outcomes. For example, researchers have used machine learning algorithms to identify patterns of brain activity associated with depression or schizophrenia. These models can help predict an individual’s risk of developing these disorders and inform personalized treatment strategies.
4. Neurofeedback Therapy
Neurofeedback is a form of therapy that uses real-time feedback of brain activity to teach self-regulation of brain function. Data science methods are employed to analyze brain signals in real-time and provide feedback to patients. By combining neuroscience principles with data science algorithms, neurofeedback therapy can be tailored to target specific brain regions or patterns associated with neurological or psychiatric conditions.
5. Brain Connectivity Analysis
Understanding how different brain regions communicate with each other is crucial for understanding brain function. Data science techniques, such as graph theory and network analysis, are used to analyze brain connectivity data derived from techniques like fMRI and EEG. This allows researchers to map the complex networks of connections within the brain and investigate how alterations in connectivity may contribute to neurological disorders.
6. Drug Discovery and Development
Data science approaches are increasingly being applied to analyze large-scale biological and neuroimaging datasets to identify potential drug targets for neurological disorders. By integrating data from genomics, proteomics, and neuroimaging studies, researchers can identify molecular pathways involved in disease pathogenesis and develop targeted therapies.
These examples demonstrate the diverse ways in which neuroscience and data science interact to advance our understanding of the brain and develop innovative approaches for diagnosis, treatment, and rehabilitation in neurological and psychiatric disorders.
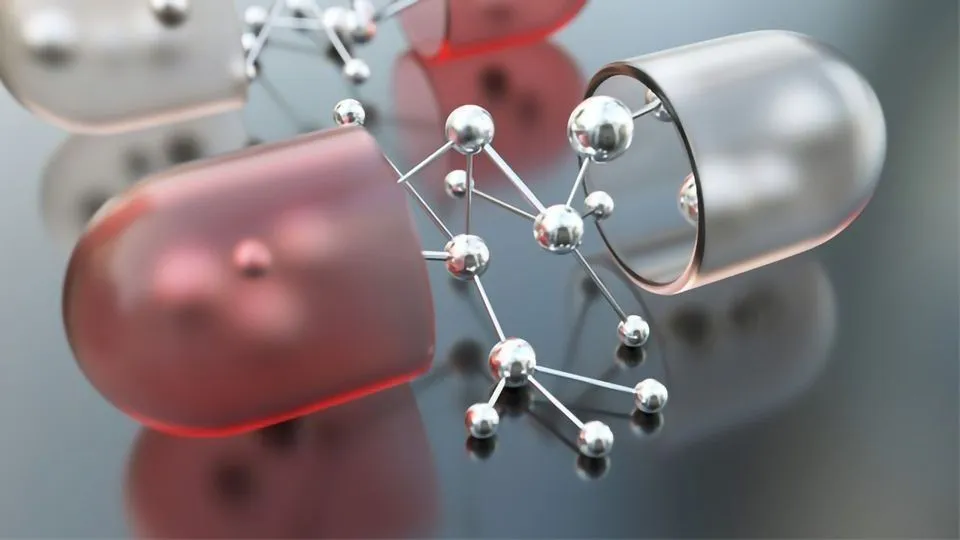
Ethical Considerations
Of course, the interaction between neuroscience and data science raises important ethical considerations. Issues like data privacy, informed consent, and the potential misuse of predictive models must be carefully considered and addressed. Researchers and practitioners must ensure that their work is conducted ethically and responsibly, with the well-being of individuals and society at the forefront.
Conclusion
In conclusion, the interaction between neuroscience and data science is a powerful partnership that holds tremendous promise for advancing our understanding of the brain and improving clinical outcomes. By combining their strengths, these two fields can unlock new insights into the complexities of the brain and develop innovative approaches to diagnosis, treatment, and prevention. As we continue to explore the dynamic interplay between neuroscience and data science, we open up new possibilities for addressing some of the most pressing challenges in brain health and beyond.