Machine Learning in Bioinformatics stands as a transformative force, reshaping the landscape of scientific inquiry. With its ability to interpret complex biological data and predict patterns, it opens doors to new discoveries and insights.
In this blog, we’ll explore how Machine Learning in Bioinformatics is revolutionizing biological research, explore its applications in biological systems, uncover the role of Deep Learning in Bioinformatics, examine how AI is utilized in this field, and ponder the future prospects it holds.
Machine Learning in Bioinformatics: A Paradigm Shift in Biological Research
Machine Learning in Bioinformatics marks a paradigm shift in biological research, empowering scientists to tackle intricate biological questions with precision and efficiency.
By leveraging computational algorithms to analyze vast amounts of biological data, machine learning enables researchers to extract meaningful patterns and insights like never before. It is driving innovation across diverse domains of biological systems.
Applications of Machine Learning in Biological Systems
- Genomic Sequencing and Analysis
Genomic sequencing, the process of determining the complete DNA sequence of an organism, lies at the heart of modern biology. Bioinformatics machine learning algorithms play a pivotal role in analyzing genomic data, aiding in gene discovery, variant calling, and phylogenetic analysis.
For example, tools like BLAST (Basic Local Alignment Search Tool) employ machine learning techniques to compare DNA sequences and identify evolutionary relationships between organisms, facilitating research in genetics and evolutionary biology.
- Protein Structure Prediction
Understanding the three-dimensional structure of proteins is crucial for understanding their functions and designing therapeutic interventions. Machine Learning in bioinformatics algorithms, particularly those based on Deep Learning architectures, have made significant strides in protein structure prediction.
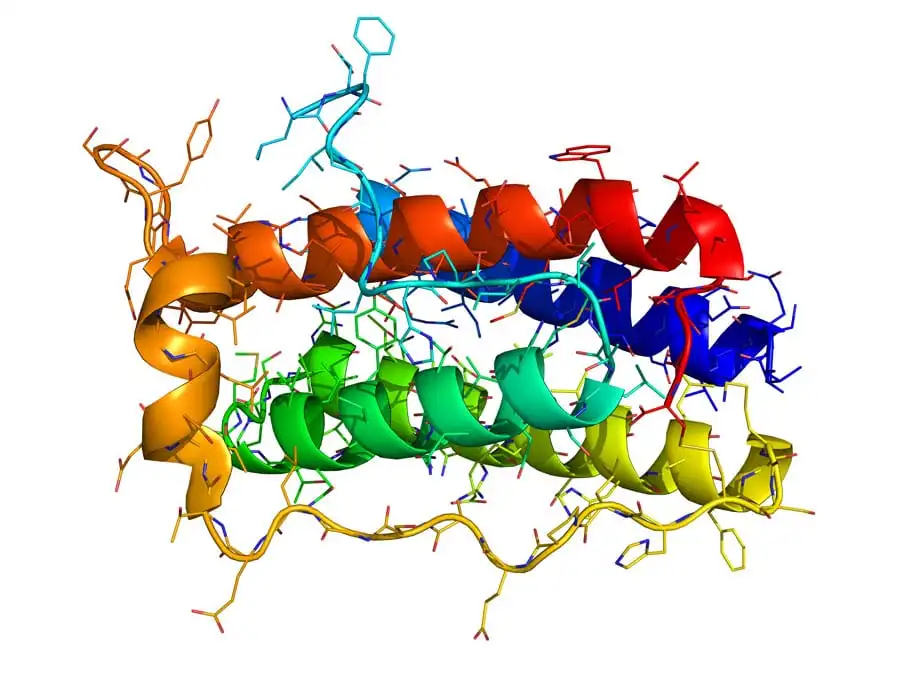
For instance, AlphaFold, a Deep Learning-based model developed by DeepMind, achieved groundbreaking accuracy in predicting protein structures, revolutionizing the field of structural biology and accelerating drug discovery efforts.
- Drug Discovery and Development
Machine Learning plays a vital role in drug discovery and development, offering computational tools to expedite the identification of potential drug candidates and understand their interactions with biological targets.
For example, virtual screening techniques employ machine learning algorithms to sift through vast chemical libraries and prioritize compounds with the greatest therapeutic potential. Such approaches streamline the drug development pipeline, reducing costs and time-to-market for novel therapeutics.
- Biomedical Image Analysis
Biomedical imaging techniques generate vast amounts of visual data, providing insights into cellular structures, tissue morphology, and disease pathology. Machine Learning algorithms excel in analyzing biomedical images, enabling tasks such as image segmentation, object detection, and disease diagnosis.
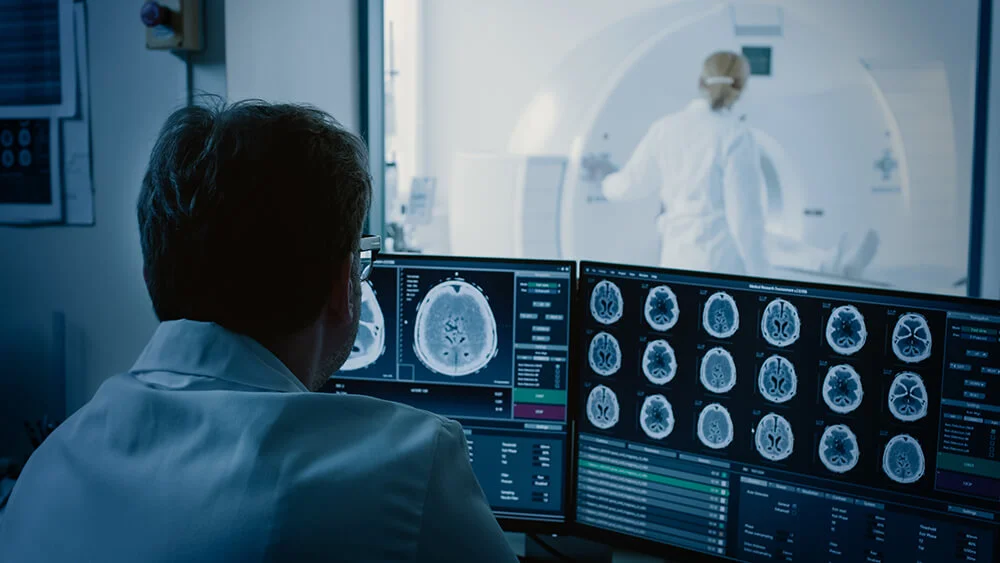
For instance, Convolutional Neural Networks (CNNs) have demonstrated remarkable performance in classifying histopathology images for cancer diagnosis, augmenting the capabilities of pathologists and improving patient outcomes.
The Role of Deep Learning in Bioinformatics
Deep Learning, a subset of machine learning inspired by the structure and function of the human brain’s neural networks, holds tremendous potential in Bioinformatics:
- Enhanced Pattern Recognition
Deep Learning models excel in capturing intricate patterns and relationships within biological data, enabling more accurate predictions and classifications. In tasks such as genomic sequence analysis and protein structure prediction, Deep Learning architectures outperform traditional machine learning approaches, harnessing the hierarchical representations learned from raw data to uncover hidden insights.
- Transfer Learning
Transfer learning, a technique where knowledge gained from pre-trained models is transferred to new tasks, offers significant advantages in Bioinformatics. Deep Learning models trained on large-scale datasets, such as ImageNet or natural language corpora, can be fine-tuned for specific biological tasks with limited labeled data. This approach accelerates model training and improves performance, especially in domains where data scarcity is a challenge.
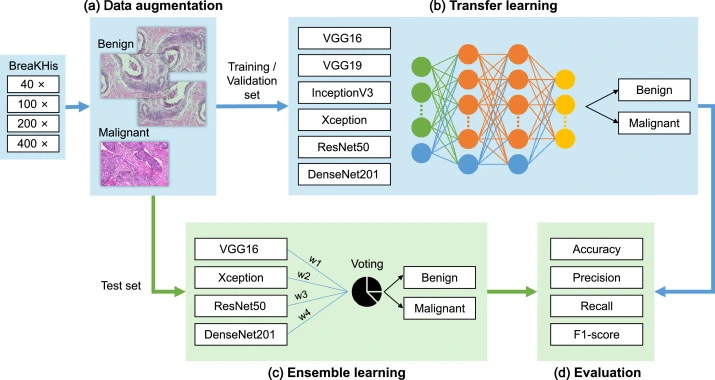
- Interpretable Representations: Shedding Light on Biological Mechanisms
Deep Learning models capable of learning interpretable representations from biological data hold promise for elucidating underlying biological mechanisms. By visualizing learned features or attention maps, researchers can gain insights into the molecular basis of diseases, drug interactions, and biological pathways. Such interpretability enhances the trustworthiness of Deep Learning models and fosters collaboration between computational biologists and domain experts.
AI Application in Bioinformatics
Artificial Intelligence (AI), encompassing machine learning, Deep Learning, and other computational techniques, plays a transformative role in Bioinformatics.
Explore about Artificial Intelligence or Data Science…which career will be best choice for you?
- Personalized Medicine
AI-driven approaches enable personalized medicine by analyzing patients’ genetic profiles and clinical data to tailor treatment strategies. Machine Learning bioinformatics algorithms identify genetic variants associated with disease susceptibility, drug response, and treatment outcomes, empowering healthcare providers to deliver precision therapies that maximize efficacy and minimize adverse effects.
For example, pharmacogenomics leverages AI to predict how individuals will respond to specific medications based on their genetic makeup, optimizing treatment selection and dosage.
Know more about how data science in medicine is revolutionizing the healthcare
- Biological Data Mining
AI techniques mine vast repositories of biological data to uncover hidden patterns, correlations, and insights that inform research and clinical practice. From analyzing gene expression profiles to modeling protein-protein interactions, machine learning algorithms facilitate knowledge discovery in diverse areas of biology.
For instance, network-based approaches utilize AI to construct biological networks from omics data, revealing intricate relationships between genes, proteins, and pathways.
- Drug Repurposing
AI accelerates drug discovery by repurposing existing drugs for new therapeutic indications through computational screening of drug-target interactions. Bioinformatics machine learning algorithms predict the efficacy and safety of drug candidates by analyzing molecular structures, biological pathways, and clinical data. By repurposing approved drugs for alternative uses, AI shortens the drug development timeline, reduces costs, and expands the repertoire of treatment options available to patients.
Future Prospects of Machine Learning in Bioinformatics
The future of Machine Learning in Bioinformatics holds immense promise, with several exciting prospects on the horizon:
- Multi-Omics Integration: Bridging the Gap between Genotype and Phenotype
Integrating data from multiple omics layers, including genomics, transcriptomics, proteomics, and metabolomics, promises a comprehensive understanding of biological systems. Machine Learning techniques capable of integrating and analyzing multi-omics data will unravel complex genotype-phenotype relationships, advancing our knowledge of disease mechanisms and therapeutic targets.
- Explainable AI: Shedding Light on Black-Box Models
As AI-driven models become increasingly sophisticated, ensuring their interpretability and transparency is crucial for trust and adoption in bioinformatics research and healthcare. Explainable AI techniques aim to elucidate the rationale behind AI predictions and decisions, enabling researchers and clinicians to understand and validate model outputs.
By providing insights into the underlying biological mechanisms, explainable AI enhances the reliability and utility of Machine Learning models in bioinformatics applications.
- Synthetic Biology: Engineering Novel Biological Systems with AI
Machine Learning algorithms are poised to revolutionize synthetic biology by designing and optimizing novel biological systems with desired functions. AI-guided design of genetic circuits, metabolic pathways, and microbial communities promises breakthroughs in biomanufacturing, bioenergy production, and environmental remediation.
By harnessing the power of AI to engineer living organisms, synthetic biology offers innovative solutions to global challenges in healthcare, agriculture, and sustainability.
In conclusion, Machine Learning in Bioinformatics is driving a paradigm shift in biological research, enabling scientists to explore the complexities of life with unprecedented precision and efficiency.
Still confused about right career choice? Explore more about 8 future careers of biological data science to look forward to in 2024