The fusion of data science and medicine has given birth to a field that holds immense potential for transforming the way we approach healthcare. Data science in medicine plays a pivotal role in deciphering complex biological information, enabling healthcare professionals to make informed decisions and deliver targeted treatments.
In this blog, we will explore how data science is utilized in medicine and pharmaceuticals with real-world examples, and gaze into the promising future it holds for mankind.
Understanding Data Science in Medicine
Data science in medicine involves the collection, analysis, and interpretation of vast datasets to derive meaningful insights that can enhance patient care, drug discovery, and overall healthcare management.
Following are the applications of data science in medicine with examples for better understanding:
1. Genomic Data Analysis:
- Role: Data science in medicine is instrumental in the analysis of genomic data, unraveling the individual’s genetic code. Explore more about how to do step by step genomic analysis in Python and R.
- Function: Algorithms and statistical models are employed to identify genetic variations, mutations, and markers that may influence disease susceptibility and treatment response.
- Significance: This allows healthcare professionals to develop a comprehensive understanding of the genetic basis of diseases, paving the way for tailored treatments based on a patient’s unique genetic profile.
- Real-World Example: The Cancer Genome Atlas (TCGA) project, a cornerstone of data science in medicine, delves into genomic data analysis. By scrutinizing the genetic makeup of various cancers, TCGA provides researchers and clinicians with invaluable insights. For instance, in breast cancer, specific genetic mutations guide the development of targeted therapies, showcasing how data science in medicine tailors treatments to individual genetic profiles.

2. Predictive Analytics for Treatment Response:
- Role: Data science in medicine facilitates predictive analytics, foreseeing how an individual or a population is likely to respond to a particular treatment.
- Function: Machine learning algorithms analyze historical treatment data, patient outcomes, and genetic information to predict the efficacy and potential side effects of specific therapies. Find out more about Machine Learning Applications in Bioinformatics.
- Significance: This predictive capability enables healthcare providers to choose treatments with higher success rates, minimizing the trial-and-error approach and reducing the risk of adverse reactions.
- Real-World Example: In the realm of data science in medicine, Oncotype DX stands out for its predictive analytics prowess. This genomic test analyzes gene expression in breast cancer tumors, predicting the risk of recurrence. By doing so, it aids healthcare professionals in making informed decisions about the necessity of chemotherapy. This real-world application minimizes unnecessary treatments and aligns with the goal of data-driven precision medicine.
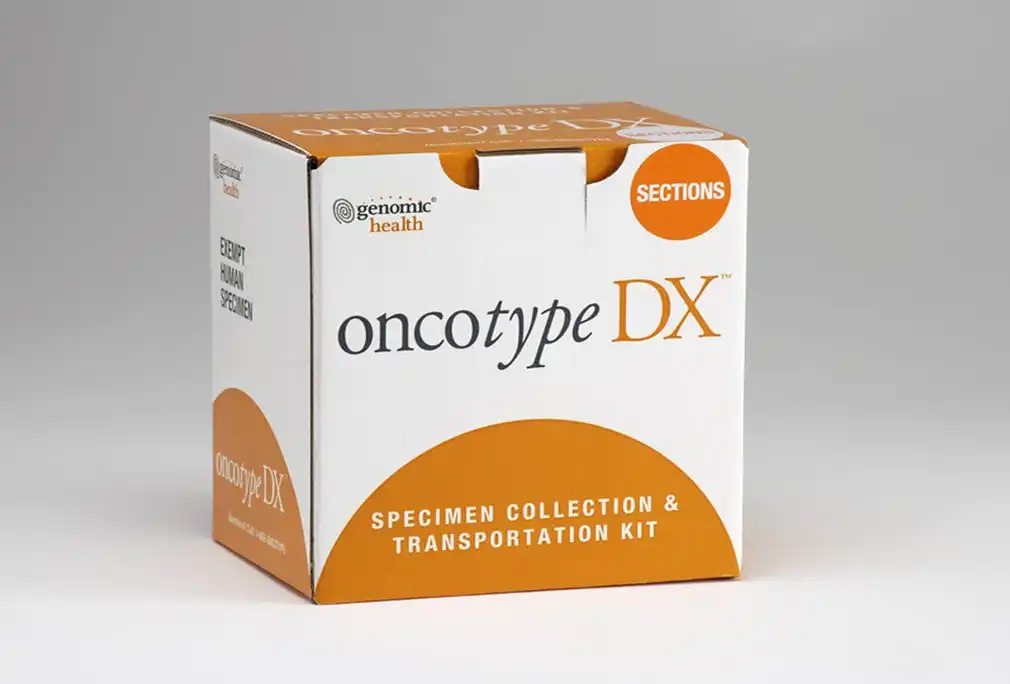
3. Integration of Multi-Omics Data:
- Role: Data science in medicine integrates multi-omics data, combining information from genomics, proteomics, metabolomics, and other -omics disciplines.
- Function: Sophisticated algorithms process and interpret diverse biological data sets, offering a holistic view of a patient’s molecular profile. Explore all types of biological data sets and their formats in data science.
- Significance: The comprehensive understanding derived from multi-omics data enhances the precision of treatment decisions, considering the intricate interplay of various biological factors.
- Real-World Example: The Human Microbiome Project epitomizes the integration of multi-omics data. By combining genomics, proteomics, and metabolomics data, the project unravels the complex interactions within the human microbiome. This holistic understanding empowers personalized interventions that target the microbiome, exemplifying how data science in medicine harmonizes diverse biological insights for improved health outcomes.

4. Electronic Health Records (EHR) Analysis:
- Role: Data science digs into the analysis of Electronic Health Records (EHR), extracting valuable insights from patient histories, diagnostic records, and treatment outcomes.
- Function: Natural language processing and machine learning algorithms mine EHR data to identify patterns, trends, and correlations relevant to personalized medicine.
- Significance: By leveraging EHR data, healthcare providers gain a real-world perspective on patient responses to treatments, aiding in the refinement of personalized treatment plans.
- Real-World Example: Data science in medicine, as exemplified by the Mayo Clinic’s initiatives, mines Electronic Health Records (EHRs) for actionable insights. The analysis of patient records, including medical histories and treatment outcomes, aids in identifying patterns associated with successful treatment responses. Find out more about Clinical Data Science as a career choice in 2024 and what 8 essential skills are required to become Clinical Data Analyst.
5. Pharmacogenomics and Drug Development:
- Role: Data science contributes significantly to pharmacogenomics, examining how an individual’s genetic makeup influences their response to medications.
- Function: Computational models predict how specific genetic variations may impact drug metabolism, efficacy, and potential side effects.
- Significance: This information guides the development of targeted drugs and dosage recommendations, ensuring optimal therapeutic outcomes tailored to individual patients.
- Real-World Example: Pharmacogenomics, a vital component of data science in medicine, played a pivotal role in the development of Trastuzumab (Herceptin) for breast cancer. Genetic markers, identified through data science approaches, guide the selection of patients who would respond positively to the drug.
6. Machine Learning for Risk Prediction:
- Role: Data science employs machine learning algorithms to predict disease risks and prognosis based on individual patient data.
- Function: By analyzing a combination of genetic, lifestyle, and environmental factors, machine learning models can forecast the likelihood of disease occurrence and progression.
- Significance: Healthcare providers can implement preventive measures and personalized interventions based on the identified risk factors, contributing to early disease detection and management.
- Real-World Example: The Framingham Heart Study utilizes machine learning algorithms for risk prediction in cardiovascular diseases. By considering an array of factors, including genetics, lifestyle, and environmental elements, the study provides personalized risk assessments. This proactive approach, empowered by machine learning, allows individuals to make informed decisions and undertake preventive measures based on their predicted risk, showcasing the practical applications of data science in medicine.
7. Real-Time Monitoring and Feedback:
- Role: Data science enables real-time monitoring of patients, continuously analyzing data streams from wearable devices, sensors, and other monitoring tools.
- Function: Algorithms process and interpret real-time health data, providing immediate feedback to healthcare professionals and patients.
- Significance: This real-time monitoring facilitates dynamic adjustments to personalized treatment plans, ensuring adaptive and responsive healthcare interventions.
- Real-World Example: Continuous glucose monitoring systems in diabetes management epitomize real-time monitoring in the context of data science in medicine. These devices, utilizing data science algorithms, analyze blood glucose levels in real-time, providing immediate feedback to healthcare professionals and patients. This dynamic feedback loop enables swift adjustments to treatment plans, ensuring personalized and responsive healthcare interventions tailored to individual needs.
Challenges and Future Prospects of Data Science in Medicine
Using data science in medicine is like embarking on a big journey with lots of possibilities, but there are challenges. We face problems, from tricky ethical questions to complicated technical stuff. It’s important to understand and solve these challenges to make the most of the amazing things data science can do in the field of medicine.
- Data Security and Privacy:
- The integration of extensive genetic and personal data raises concerns about privacy and the potential misuse of sensitive information.
- Access and Equity:
- Ensuring widespread access to personalized medicine is a challenge, as it requires advanced technologies and may be costly.
- Interdisciplinary Collaboration:
- Successful implementation of personalized medicine requires collaboration among diverse fields, including genetics, informatics, and healthcare.
The trajectory of data science in medicine is set to evolve even further, promising groundbreaking developments that will reshape the healthcare landscape.
Integration of Artificial Intelligence (AI):
- AI algorithms will play a more significant role in disease prediction, diagnosis, and treatment planning.
- Example: AI-powered chatbots assisting patients in symptom assessment and providing initial healthcare recommendations.
Advancements in Precision Medicine:
- Precision medicine will become more precise with the integration of advanced technologies, offering tailored treatments at an unprecedented level.
- Example: CRISPR technology enabling precise gene editing for therapeutic purposes.
Interconnected Healthcare Ecosystem:
- Data sharing among different healthcare entities will foster collaboration and improve the overall quality of care.
- Example: Interconnected Electronic Health Record (EHR) systems allowing seamless sharing of patient information among healthcare providers.
In summary, when data science joins forces with medicine, it’s changing healthcare in really big ways. We can see this happening with real examples that show how it’s helping with personalized medicine, finding new drugs, and managing healthcare better. We already see benefits like better diagnosis, improved treatments, and smarter healthcare management.
However, it’s not all smooth sailing. There are challenges we need to tackle, like making sure we handle data ethically and dealing with technical complexities. But if we overcome these challenges, the future looks even brighter. With data science in medicine, we’re on a path to a healthier and better tomorrow.