In the world of science and medicine, there’s something incredibly valuable: data. But it’s not just about numbers or information. It’s about using that data to learn about living things. Bio data analytics helps us do this. It’s like using a special tool to understand the information hidden in biological data.
What is Bio Data Analytics?
At its core, bio data analytics is the art and science of translating biological data into meaningful insights. It helps us find patterns and answers in the data we collect by employing advanced computational techniques, statistical analysis, and domain expertise. Bio data analytics transforms raw data into actionable knowledge. This can help us learn about diseases, find new medicines, and make better choices for our health.
What is the Importance of Bio Data Analytics?
Diversity and the complexity are two main challenges researchers and healthcare professionals face when dealing with various types of biological data. In such scenario, bio data analytics serves as the guiding light, helping us navigate through the complexity of biological systems to uncover hidden patterns, identify biomarkers, and make sense of the data.
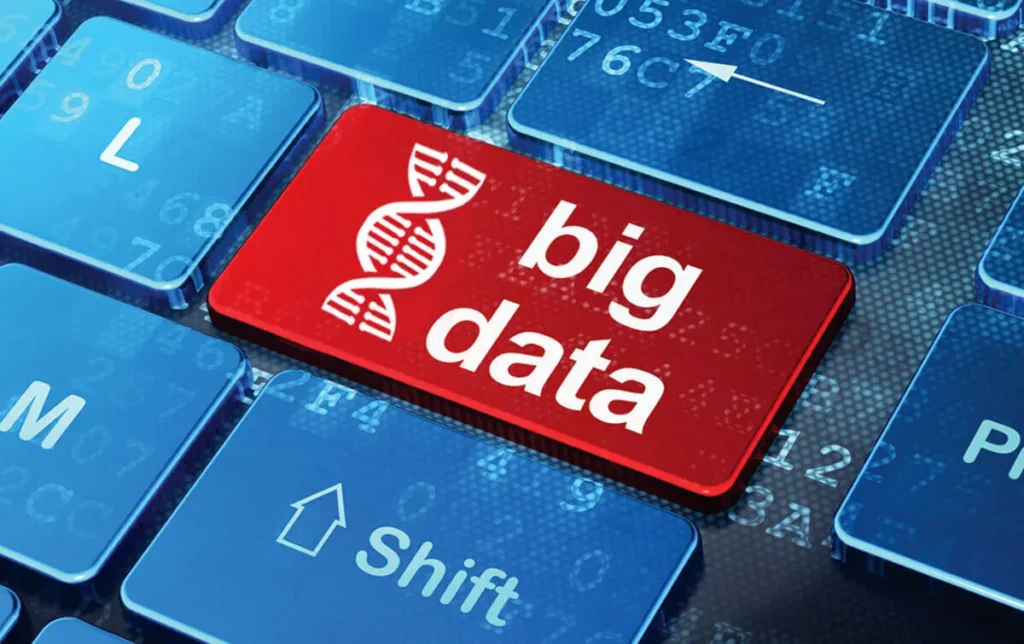
What are Applications of Bio Data Analytics?
Precision Medicine:
One of the most promising applications of bio data analytics is in the field of precision medicine. By analyzing an individual’s genetic makeup, lifestyle factors, and environmental exposures, doctors can tailor treatments to each patient’s unique characteristics.
For example, researchers at the Mayo Clinic used bio data analytics to identify genetic mutations associated with drug responses in patients with rheumatoid arthritis, paving the way for personalized treatment strategies.
Drug Discovery:
The process of discovering new drugs is both time-consuming and expensive. Bio data analytics has the potential to revolutionize this process by accelerating the identification of potential drug targets and predicting drug responses.
For instance, scientists at the University of Toronto used machine learning algorithms to analyze genomic data and identify novel drug candidates for the treatment of antibiotic-resistant infections.
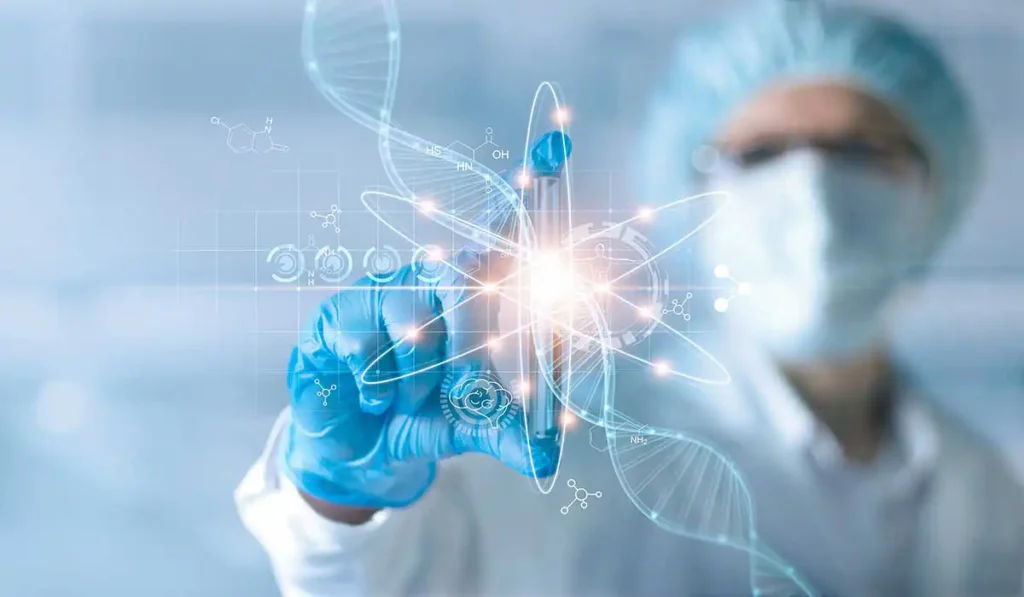
Disease Diagnosis and Prognosis:
Early detection and accurate diagnosis are critical for effectively managing diseases. Bio data analytics can aid in the early detection of diseases such as cancer, diabetes, and Alzheimer’s disease by analyzing biomarkers and imaging data. Additionally, bio data analytics can help predict disease progression and inform treatment decisions.
For example, researchers at Stanford University developed a machine learning algorithm that analyzes electronic health records to predict sepsis, a life-threatening condition, before it occurs.
Genomics and Personalized Healthcare:
The advent of next-generation sequencing technologies has ushered in a new era of genomic medicine. Bio data analytics plays a crucial role in analyzing genomic data to uncover genetic variants associated with disease risk and drug response. By integrating genomic data with clinical information, researchers can develop personalized healthcare strategies tailored to each individual’s genetic makeup.
For instance, the All of Us Research Program, sponsored by the National Institutes of Health, aims to collect and analyze genomic and health data from one million or more participants to advance precision medicine.
What Tools and Techniques are used in Biological Data Analytics?
Machine Learning:
Machine learning algorithms have become indispensable tools in bio data analytics due to their ability to extract patterns and relationships from large datasets. From classification and regression to clustering and dimensionality reduction, machine learning techniques can be applied to a wide range of biological data types, including genomic, proteomic, and imaging data.
Data Visualization:
Data visualization is essential for conveying complex biological information in a comprehensible manner. Tools such as heatmaps, scatter plots, topological analysis and network diagrams enable researchers to explore and interpret large-scale biological datasets effectively.
For example, the UCSC Genome Browser provides a user-friendly interface for visualizing genomic data, including gene annotations, sequence alignments, and epigenetic modifications.
Statistical Analysis:
Statistical methods are the foundation of bio data analytics, providing the framework for hypothesis testing, parameter estimation, and uncertainty quantification. From simple t-tests and ANOVAs to more advanced techniques such as linear regression, bivariate data analysis, partial correlations and other statistical analysis enables researchers to draw reliable conclusions from biological data.
For example, researchers at the Broad Institute of MIT and Harvard developed the Gene Set Enrichment Analysis (GSEA) method, which uses statistical techniques to identify biological pathways enriched in gene expression data.
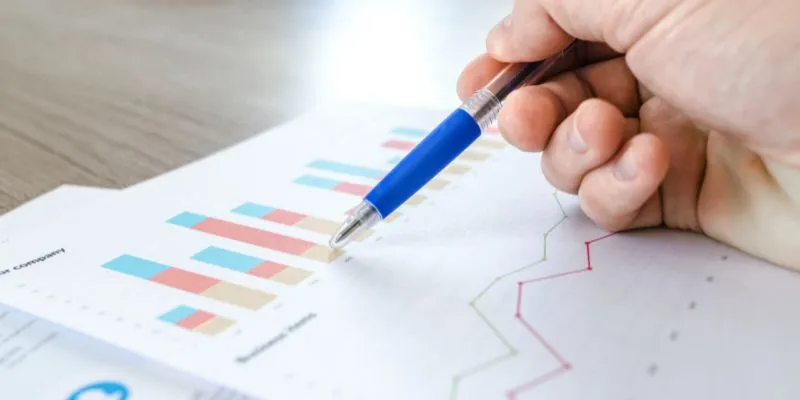
What are the Challenges in Bio Data Analytics?
Data Quality and Quantity:
One of the foremost challenges in bio data analytics is ensuring the quality and quantity of the data. Biological datasets are often noisy, incomplete, and heterogeneous, posing significant challenges for analysis and interpretation. Furthermore, the sheer volume of data being generated by high-throughput technologies such as next-generation sequencing and mass spectrometry exceeds the capacity of traditional data analysis methods.
Addressing these challenges requires the development of robust quality control procedures, data integration techniques, and scalable analysis pipelines.
Interdisciplinary Collaboration:
Bio data analytics is inherently interdisciplinary, requiring collaboration between biologists, statisticians, computer scientists, and healthcare professionals. However, bridging the gap between these diverse disciplines can be challenging due to differences in terminology, methodology, and research culture. Effective interdisciplinary collaboration requires clear communication, mutual respect, and a shared commitment to addressing complex biological questions.
Initiatives such as the BD2K (Big Data to Knowledge) program, sponsored by the National Institutes of Health, aim to foster collaboration and innovation in bio data analytics through interdisciplinary training programs and research grants.
Ethical Considerations:
As bio data analytics becomes increasingly pervasive in healthcare and research, ethical considerations surrounding data privacy, consent, and ownership are of paramount importance. The widespread availability of personal health information and genomic data raises concerns about data security, confidentiality, and potential misuse. Moreover, the commodification of biological data raises questions about the equitable distribution of benefits and risks.
Addressing these ethical concerns requires a multifaceted approach involving policymakers, researchers, industry stakeholders, and the public. Initiatives such as the Global Alliance for Genomics and Health (GA4GH) aim to develop international standards and guidelines for ethical data sharing and use in bio data analytics.
Conclusion
In conclusion, bio data analytics holds immense promise for advancing our understanding of biology and improving healthcare outcomes. With the help of computational techniques, statistical analysis, and domain expertise, bio data analytics enables researchers and healthcare professionals to unlock the secrets hidden within biological data.
However, realizing this potential requires overcoming significant challenges related to data quality, interdisciplinary collaboration, and ethical considerations. By addressing these challenges and embracing the opportunities afforded by bio data analytics, we can usher in a new era of precision medicine, personalized healthcare, and scientific discovery.